Understanding the Concept of Bounding Box in Data Annotation
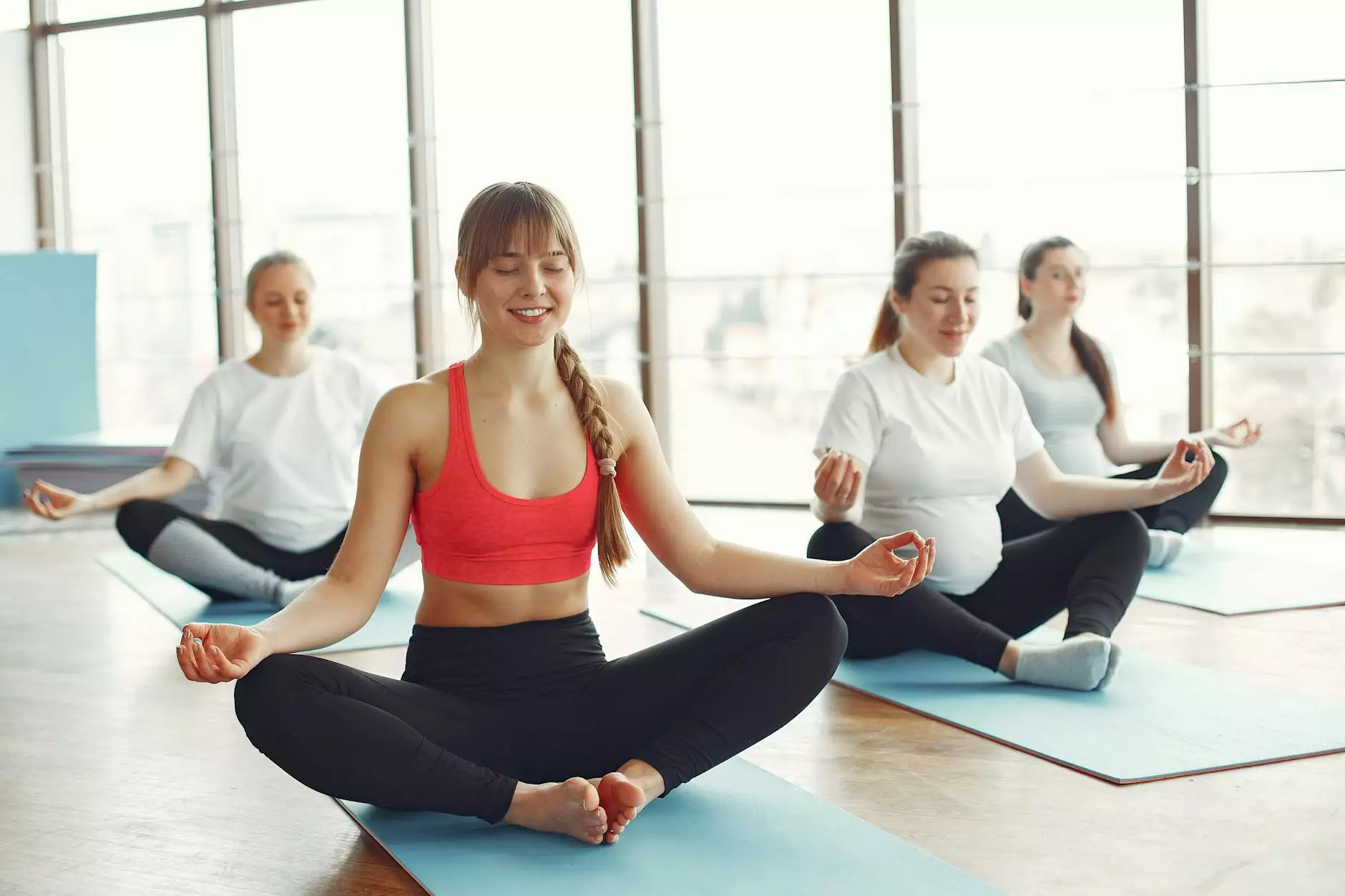
The term bounding box often emerges in discussions around data annotation, particularly in the realm of machine learning and artificial intelligence. In this detailed article, we will explore the importance of bounding boxes, their applications, and how platforms such as Keylabs.ai utilize this concept to enhance the annotation process.
What is a Bounding Box?
A bounding box is defined as a rectangular box that is used to represent the position of an object within a larger image or video frame. Its primary purpose is to mark the presence and location of objects, providing a simple yet effective way for machines to understand visual data.
Bounding boxes are primarily utilized in computer vision tasks, often serving as a starting point for more complex object detection and recognition algorithms. By identifying and capturing objects of interest within images, data scientists can train algorithms to recognize similar patterns in new visual input.
The Role of Bounding Boxes in Machine Learning
In the context of machine learning, the annotation of image data plays a critical role in the efficacy of predictive models. When we talk about bounding boxes, we are generally referring to the following applications:
- Object Detection: Bounding boxes allow models to pinpoint and classify objects within images, making it essential for tasks such as facial recognition, license plate recognition, and more.
- Image Segmentation: In image segmentation tasks, bounding boxes can serve as a preliminary step to delineate object boundaries before applying more sophisticated segmentation techniques.
- Activity Recognition: Bounding boxes help in tracking objects' movements within video frames, enabling accurate activity recognition over time.
Through bounding boxes, machines learn to identify, evaluate, and interact with the visual world, advancing applications in autonomous vehicles, robotics, and security surveillance.
How Bounding Boxes Enhance Data Annotation Process
Data annotation plays a pivotal role in creating datasets that are critical for training machine learning models. Here's how bounding boxes enhance this process:
- Efficient Annotation: By defining the boundaries of each object, query responses are faster, and taps into data labeling become more efficient.
- Precision: Bounding boxes provide a structured approach to labeling images, ensuring that the annotations are precise and uniform across datasets.
- Scalability: As image datasets grow larger, having a consistent bounding box structure allows for easier management and scalability.
Bounding Box Types: A Closer Look
There are various types of bounding boxes that can be used depending on the application's needs:
- 2D Bounding Boxes: These boxes define an area in two dimensions (width and height), commonly used in typical image annotations.
- 3D Bounding Boxes: Often used in autonomous driving scenarios, these boxes encompass objects in three-dimensional space.
- Rotated Bounding Boxes: Unlike standard rectangles, rotated bounding boxes are used to enclose objects that are not axis-aligned, providing a more accurate representation of their contours.
Benefits of Using a Bounding Box in Data Annotation Platforms
Choosing a data annotation platform that effectively implements bounding boxes can substantially impact your project’s success:
- Improved Accuracy: Leveraging bounding boxes enhances annotation accuracy, leading to better training results for machine learning models.
- Cost-Effectiveness: High-quality annotations save businesses time and reduce the costs associated with reworking or re-labeling datasets.
- Enhanced Collaboration: A robust data annotation platform allows multiple workers to collaborate more efficiently on annotating large datasets.
Keylabs.ai: Your Partner in Data Annotation
At Keylabs.ai, we offer a comprehensive data annotation tool that utilizes bounding boxes to streamline the annotation process. Our platform brings together advanced technology and expert annotators to deliver high-quality labeled datasets.
- User-Friendly Interface: Our intuitive interface ensures that users can easily create bounding boxes and label objects without any steep learning curve.
- Scalable Solutions: Regardless of the project's size, our platform can scale with your needs, allowing for the annotation of thousands of images with consistent quality.
- Expert Guidance: Our team of data experts is always available to provide support and ensure that your annotation process achieves the desired outcome.
Conclusion
In conclusion, the concept of bounding box is integral to the field of data annotation, significantly influencing the performance of machine learning applications. By adopting efficient and precise bounding box annotations through platforms like Keylabs.ai, organizations can enhance their data collection, achieve better model performance, and ultimately drive innovation across various industries.
As the demand for high-quality data labeling continues to grow, understanding the value of bounding boxes and leveraging advanced data annotation platforms will be critical in staying ahead in the competitive landscape of artificial intelligence.
Get Started with Keylabs.ai Today
If you're ready to elevate your data annotation processes to the next level, explore how supportive solutions like Keylabs.ai can assist you. Join us to take advantage of our powerful annotation tools tailored to your specific needs!